Who would have thought that my sweaty palms could ever be relevant for a study … I am Anna, a working student at Fraunhofer IIS, and that’s what I’m thinking to myself as I’m sitting wired up on a chair in a set-up box with dim lighting. Two electrodes are sticking to my hand and chest. Several cameras are focused on me, making me uneasy, but I get used to it. A small clip on my finger is measuring my blood volume, while wires on my palm are measuring my sweat. I’ve never been in a situation like this before. But I’m sitting in the exposure cabin at Fraunhofer IIS, taking part in a study and currently experiencing first-hand and on my own body what measurable modalities can be included in a study.
When I heard the research team was looking for volunteers for a study on emotion analysis, I was very curious. As a newbie to affective computing, I couldn’t quite imagine how they would analyze emotions, which measurable modalities they would use, and what the exact procedure would be. I only knew I would be looking at various pictures designed to evoke different emotions. That’s why I decided to take part in this study.
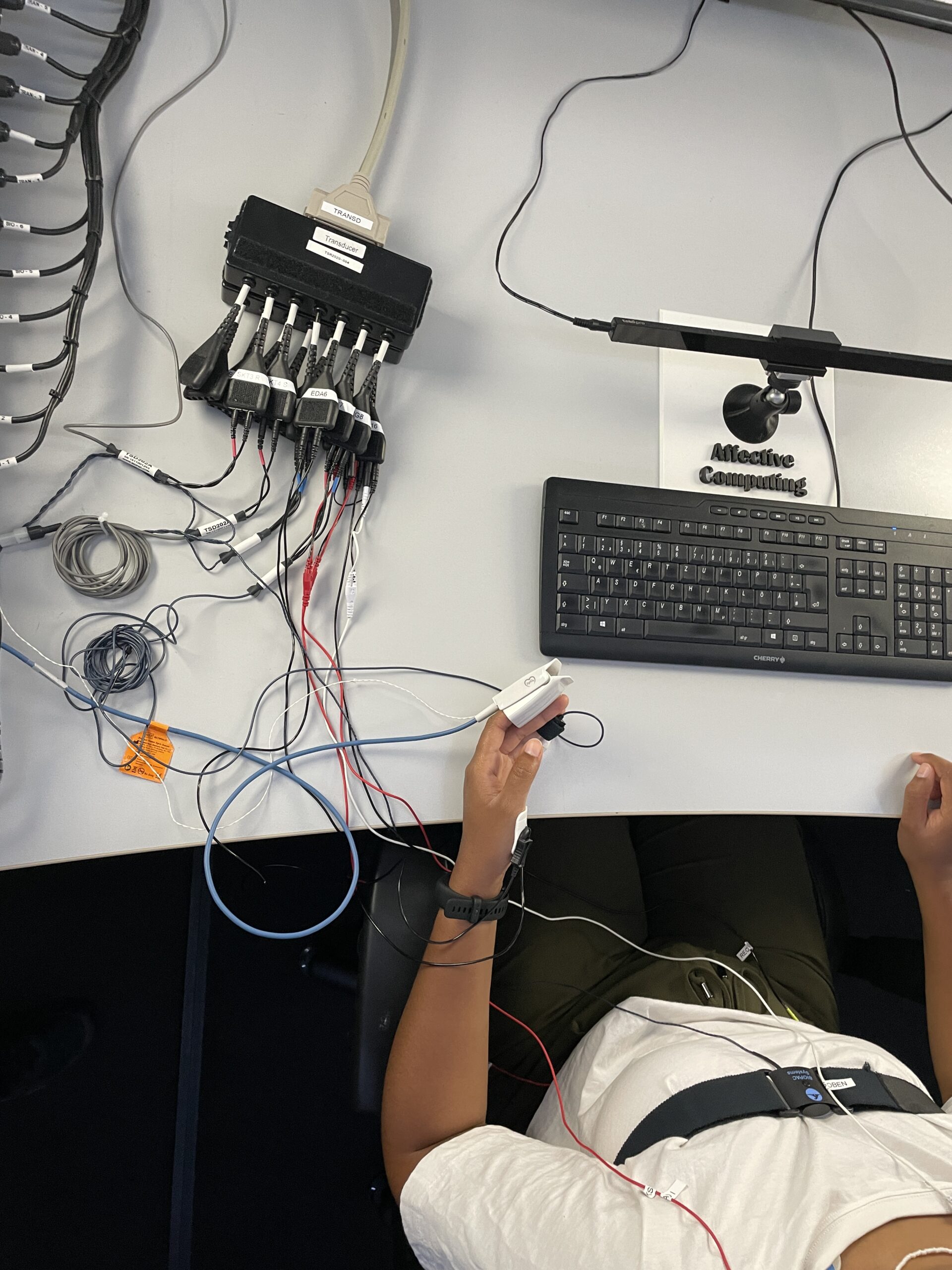
About the study
Researchers conducted an arousal study to build an all-encompassing, multimodal, and commercially usable affective computing database. They annotated a dimensional (valence-arousal) and a categorical emotion model according to Ekman 1992. The aim was to create a database for AI algorithm training, while eliminating issues like high label noise in existing databases.
In the study, modalities from different systems (industrial cameras, consumer hardware such as Garmin or Tobii, and laboratory systems such as BIOPAC), as well as the events for triggering arousal/valence, were synchronized in time with each other, and the data was recorded. This is crucial for multimodal evaluations, ensuring error-free correlation of signals from different measurement modalities. This enables understanding trigger effects, addressing latencies between modalities, and providing high-quality training for affective computing algorithms.
In addition, six different angles captured the filming, making these data sets also relevant for video processing. This data helps AIs to become robust with lateral perspectives as well. The data also enable head pose estimation, which recognizes the position of the face in relation to the camera. Such data can aid AI in digital shop windows and gesture recognition areas.
If you’re looking to get an introduction into the setup and measurement modalities of the exposure cabin, our focus article might come in handy. You can also delve into the relevant psycho-physiological signals, by reading our Emotion Analysis 101 deep dive.


Apart from the study’s scope, the exposure cabin can also be useful for safety-relevant industries, for example when assessing cognitive load in autonomous driving. Fraunhofer IIS has an extra driving simulator for this purpose. Another area where affective states play a major role, especially those that may not be directly perceptible to the human eye, is medicine. Measuring psycho-physiological reactions can be of great importance during patient anamnesis, for example.
To measure or not to measure
When I first heard about a study taking place in the exposure cabin, I had no idea what it would look like. I was thinking much more of a shoe box. It wasn’t until I entered the room that I realized it was actually a 2 x 3 meter testing space. I also learned, that the box is a modular concept. This means researchers can scale and select measurable modalities according to the study’s limitations and requirements. The decision about what exactly to measure must be made carefully. For example, if test subjects need to move a lot, as in a sports study, researchers must take this into account. The same applies if subjects cannot move, e.g., due to physical limitations.
But let’s get back on track. Before taking part in this study I wasn’t even aware of the signals researchers can measure in a person. There are quite a few, some of which I wouldn’t have thought you could measure. For example, the oxygen content of blood. So let’s take a closer look at the different measurable modalities in their categories.

Biosignals:
- EMG: Measures muscle contraction (different significance depending on the selection of the muscle).
- Oxygen: measures the oxygen in the blood during excitation.
- EDA: Measures skin perspiration using a current that flows unnoticed by the subject over the skin surface (notices resistance due to fluid accumulation).
- Respiration: Measured with a belt over the chest.
- ECG: measurement of the heart muscle using electrodes on the chest (e.g., pulse measurement).
- HRV: measures the regularity of heartbeats.
- PPG: X-rays the blood using LED technology and measures changes in blood volume.
- EGG: measures the contraction of the digestive tract.
- EEG: Current measurements of the neurons in the brain, measured superficially.
Videosignals:
- Thermal camera: measures temperature without contact, so one can also use it in poor lighting conditions.
- Eyetracker: measures saccades (how fast the pupil moves back and forth) and pupillometry (how much the pupil is open due to lighting effects during arousal and where exactly the test person is looking).
In today’s study setup, it’s a selection of these signals that are being measured. One of them is the EDA, measuring the skin perspiration, which is what got me worried about my sweaty hands.
Emotional rollercoaster: Evaluating feelings — one picture at a time
During the measurement, I was asked to look at 30 pictures in total. After every picture, I used a scale and a slider to indicate whether my feelings were positive or negative, along with the level of arousal they provoked in me. Arousal refers to the degree of physiological activation or excitement that an emotion can evoke.
Then, I had to document in further categories which emotions, such as sadness or joy, the picture triggered in me. After each picture, I had to wait 30 seconds to be able to move on to the next one in a neutral state. In total, the measurements in the box took about an hour.
After completing study, it doesn’t take me long to get unwired again. In the end, I’m just glad I wasn’t too nervous so my hands didn’t actually sweat that much.
Spoilt by choice: It doesn’t have to be that hard
Now that I have participated in the arousal study myself and learned more about emotion analysis, I still find it astonishing how many signals researchers can utilize to detect affective states. The above listed aren’t even all the signals we can measure at Fraunhofer IIS. It is possible to expand the selection through cooperation with our scientific partners. If you have a study idea and are trying to decide what to measure, our experts are here to assist you in making the best choice. Feel free to reach out and discuss your needs.
Image copyright: Fraunhofer IIS /Anna Chiwona
Add comment