Since the beginning of the energy transition in the electrical power system in Germany, the development has been going toward decentralization. Contrary to the legacy power system with big central power plants, power systems with a high share of renewables have a decentralized topology with distributed power generation. In addition, storage units are becoming more dominant, matching the non-dispatchable renewable power generation to the power consumption. Energy storage systems, by nature bringing in a bidirectional power flow, make the operation of distributed power generation more complex. This complexity necessitates a careful consideration of operational strategies and sizing. At Fraunhofer IWES, we are exploring the feasibility of simultaneously optimizing the sizing and operation of local energy systems.
Moreover, flexibility in system selection is vital, as we aim to optimize both sizing and operation across various energy systems under different regulatory frameworks and boundary conditions. This approach allows for tailored solutions that can adapt to specific regional requirements, regulatory standards, and technologies, ensuring that each local energy system is both efficient and resilient in meeting energy demands. By integrating flexibility into our analysis, we can better address the diverse challenges posed by the transition to a decentralized and renewable-based energy landscape.
What are local energy systems?
The shift in the power system towards a decentralized system with distributed power generation and storage makes it necessary to have a new view of the power system as interconnected local energy systems. A local energy system is a collection of distributed energy resources, such as renewable energy sources, energy storage systems, and local energy loads.
The local energy system acts as a single unit, making it easier to manage and optimize energy use locally. It should be designed to interact efficiently with other local energy systems and contribute to the overall stability of the electrical grid. A local energy system can operate independently (like a microgrid) when necessary, allowing for flexibility based on system needs. It can function across various voltage levels (low, medium, high), and it can utilize different types of energy, including chemical (like fuels), electrical (like electricity), and thermal (like heat). In summary, a local energy system integrates various energy resources and loads to enhance local energy management and support grid stability.
How can local energy systems be sized using the OPTILESSi toolchain?
To size a local energy system using the OPTILESSi toolchain, in the Fraunhofer IWES´ project OPTILESSi a model is formed based on the selection of components, allowing for a high degree of adaptability to various configurations. By choosing specific components, the model dynamically adjusts, reflecting the unique characteristics and energy balances of the selected elements. The model can include generation units such as wind turbines and photovoltaic arrays, a battery storage system or a hydrogen storage tank, as well as conversion units such as an electrolyzer and a fuel cell that reconverts the stored hydrogen back into electricity when required. Furthermore, a selection can be made whether to include a grid connection or to focus solely on self-consumption of the generated electricity and hydrogen to meet local demand. The configuration options are illustrated in Figure 1.
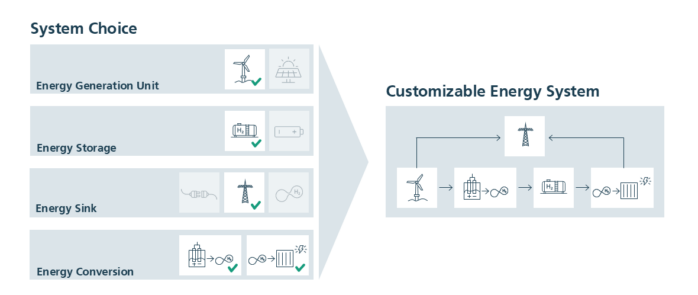
After the system has been defined, the next step is to establish the framework conditions. This includes specifying technical parameters, project characteristics, and energy demands. Additionally, the objectives of the investigation can be chosen. For instance, one objective may be economic optimization, which could involve participation in the energy market. Other compensation models, such as ancillary services or government subsidies, can also be explored. Alternatively, the focus can be directed toward local energy supply, where reliability constraints are set to ensure that demand is consistently met. The model will then utilize data from energy markets, weather conditions, or local grid status to inform its calculations. It may also take into account current regulatory requirements to ensure compliance. This comprehensive approach allows for a well-rounded analysis tailored to the specific needs and goals of the local energy system.
Once everything has been specified, model-based optimization takes place. For the model to be linear, it is assumed that efficiencies for all energy devices remain constant across different operating conditions and that the energy and power capacities of the devices do not change throughout the project’s lifecycle. This linear framework is important as it enables quicker and more straightforward optimizations over long project durations. Operational constraints ensure that all operation variables remain within defined limits and that power inputs and outputs respect maximum and minimum operational thresholds.
The sizes that need to be determined are the rated power or capacity of the components that have been selected. In addition to the optimized sizes, the model also outputs optimized operation profiles that illustrate the optimal performance trajectories of all components. These profiles are designed to ensure that the energy demands or target objectives are effectively met. By analyzing these operation profiles, stakeholders can understand how to best operate each component over time, maximizing efficiency and aligning with the specified goals of the local energy system. Additionally, key performance indicators (KPIs) are generated to assess various aspects of the system, including economic performance, adherence to supply security, and overall utilization of the system.
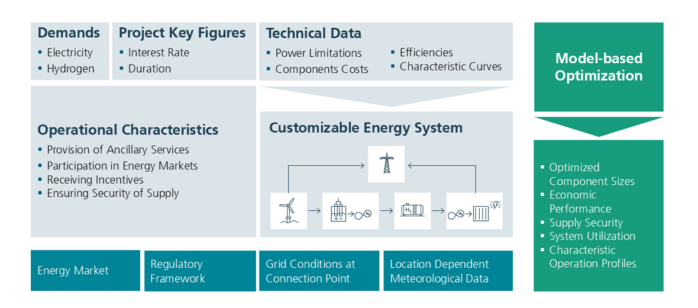
How to optimize the operation and size of a local energy system at the same time?
The model’s abstraction level, constraints, and objective function are structured in a way such that a linear programming (LP) approach is feasible, allowing the problem to be solved using an LP solver. This type of problem requires transforming the problem into a matrix formulation, expressed as minimizing a function subject to equality and inequality constraints, and bounds on the decision variables.
The decision variable vector encompasses both operational and sizing variables. The operational variables are the storage units charging and discharging and the power profiles of the generation and conversion units. All operational variables have a specific value for every sampling time, i.e., every 60 minutes of a year. Discretizing the derivatives using the Euler forward method allows recursive equations for the system states to be established, thus the formulation of the state equations at discrete-time intervals is straightforward.
Inequality constraints are added to ensure that the states remain within specified limits. This leads to multiple inequality constraints, including those related to the state of charge and power inputs. Additionally, lower and upper bounds are defined to limit the search space for the sizing variables based on user-defined choices.
Example of a local energy system optimization
This example showcases a local energy system that includes a wind turbine, an electrolyzer, a hydrogen storage tank, a fuel cell, and a grid connection. The system participates in the day-ahead EPEX market, receives an EEG-subsidy in alignment with the German regulatory framework, and provides congestion management. This means that the system prioritizes storing electricity when transmission capacity is constrained. The objective of the optimization is to maximize revenues while keeping life cycle costs (LCC) at a minimum, thereby enhancing the economic performance of the system. Figure 3 illustrates the approach taken, as well as the optimized sizes of the components.
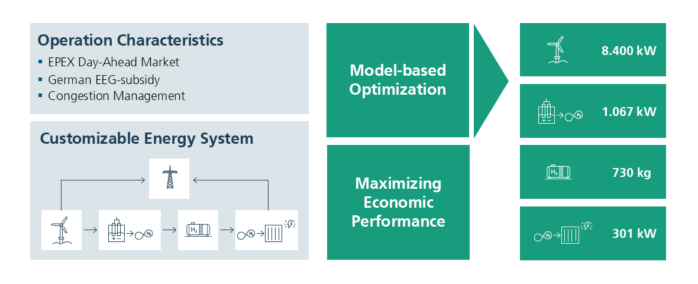
Figure 4 illustrates the operational profiles of the electrolyzer, the fuel cell, and the wind turbine. The electrolyzer is utilized when either grid feed-in is limited due to grid congestion or when the trend of the electricity prices in the EPEX market creates a more favorable scenario for reconverting stored hydrogen back into electricity at a later time.
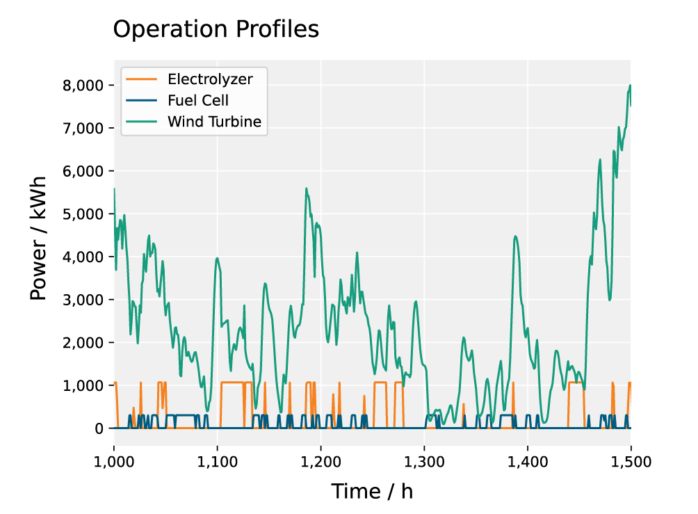
In conclusion, the OPTILESSi toolchain enables the sizing of a local energy system that can be flexibly assembled for various applications and regulatory conditions, aligning with the energy transition toward decentralization and increased renewable energy integration and the challenges of grid stability that come with it. By considering diverse prerequisites, regulatory frameworks, electricity markets, and use cases, the system can effectively optimize a wide range of applications, ranging from economic analyses to stability evaluations and supply security assessments.